Why Understanding AIOps Challenges and Solutions Matters
AIOps challenges and solutions are critical topics for modern IT teams navigating the complexities of evolving technological landscapes. As organizations adopt artificial intelligence for IT operations, they encounter hurdles such as data silos, security vulnerabilities, and skill gaps. Understanding these challenges is the first step toward creating effective strategies that ensure successful AIOps implementation.
By addressing issues like tool sprawl, integration with legacy systems, and alert noise, businesses can unlock the transformative potential of AIOps. This guide explores common obstacles and provides actionable solutions to help organizations streamline operations, enhance decision-making, and drive continuous innovation.
Key Technological Components
AIOps is a groundbreaking technological methodology that uses artificial intelligence to reshape IT operations management. It is built upon three fundamental technological pillars:
- Big Data Analytics: Manages and analyzes large-scale operational data.
- Machine Learning: Supports advanced pattern recognition and predictive insights.
- Artificial Intelligence: Facilitates automated decision-making and efficient problem-solving.
Key Features and Benefits of AIOps
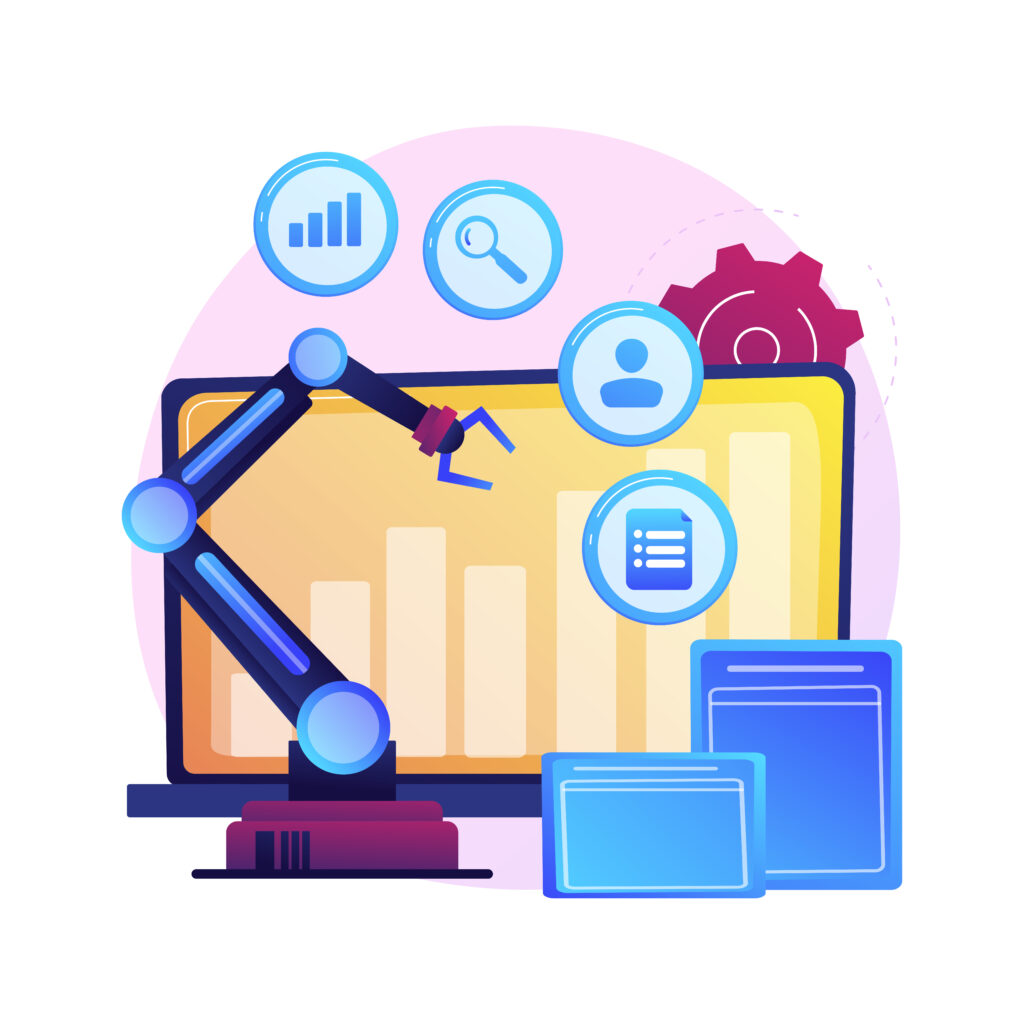
Operational Capabilities
- Automated Problem Resolution:
AIOps automates the detection and resolution of complex IT operational issues, minimizing the need for manual efforts and significantly shortening response times.
- Advanced Data Processing:
Utilizes big data analytics to process and analyze vast amounts of operational data from various digital platforms and systems.
Technological Innovations
- Machine Learning Integration:
Employs advanced machine learning algorithms to enhance operational insights and refine predictive capabilities continuously.
- Proactive Monitoring:
Uses predictive analytics to identify potential system issues early, helping to prevent service interruptions.
- Real-time Anomaly Detection:
Delivers immediate detection of system anomalies and performance irregularities.
Performance Optimization
- Network Performance Enhancement:
Boosts overall network performance through smart diagnostics and automated optimization techniques.
- Incident Resolution Acceleration:
Cuts critical incident resolution times by up to 66%, greatly enhancing operational efficiency.
- Predictive Maintenance:
Facilitates preventive maintenance by predicting potential system failures and performance issues in advance.
Operational Intelligence
- Multi-layer Technology Platform:
Integrates multiple technological elements to develop robust and comprehensive operational intelligence systems.
- Cross-platform Compatibility:
Ensures seamless functionality across various IT environments, including cloud, on-premises, and hybrid infrastructures.
Strategic Advantages
- Cost Reduction:
Lowers operational costs by automating intricate IT management tasks.
- Resource Optimization:
Enhances IT resource allocation and management with the help of intelligent analytics.
- Scalable Architecture:
Delivers adaptable solutions capable of evolving with technological advancements.
Advanced Capabilities
- Intelligent Insights Generation:
Converts raw data into actionable and strategic insights for informed decision-making.
- Security Enhancement:
Strengthens cybersecurity through advanced monitoring and threat detection capabilities.
- Continuous Learning:
Utilizes adaptive machine learning models that evolve and enhance performance over time.
Comprehensive Management
- Event Correlation:
Analyzes and connects intricate event patterns across diverse technological systems.
- Automated Workflow Management:
Optimizes IT operational workflows using intelligent automation techniques.
- Customer-Centric Operations:
Facilitates responsive and adaptive IT services tailored to meet customer needs.
The Challenges of Implementing AIOps
Skills and Talent Gap:
A significant challenge for IT organizations is the skills gap. For instance, many teams struggle to transition from manual processes to automated workflows, and they often find it difficult to evaluate and utilize AIOps solutions effectively. Therefore, addressing this issue requires investments in continuous learning, along with implementing training programs and hiring specialized AIOps professionals.
Data Quality and Integration:
Legacy systems and data silos hinder the collection and correlation of high-quality telemetry, resulting in fragmented IT infrastructure views. AIOps’ success depends on comprehensive strategies for standardizing, validating, and integrating data across diverse monitoring tools.
Security Risks:
The integration of AIOps tools can introduce new cybersecurity vulnerabilities. Organizations must implement robust encryption, access controls, and ensure compliance with standards like GDPR while thoroughly assessing the security protocols of AIOps platforms.
Complexity of IT Infrastructure:
Modern IT environments include vast arrays of endpoint and IoT devices, making monitoring and optimization challenging. AIOps solutions must effectively correlate and contextualize diverse data types to manage this complexity.
Data Volume Management:
Traditional systems struggle with processing and analyzing massive data volumes. Enterprises need production-grade pipelines to efficiently collect, clean, label, and analyze large-scale data.
Anomaly Detection Limitations:
Budget and Cost Constraints:
High initial implementation costs pose a barrier for many organizations. Pilot programs can help demonstrate ROI and pave the way for broader adoption.
Tool Sprawl and Redundancy:
Using multiple monitoring tools can lead to redundancy and increased costs. Consolidating functionalities and simplifying the IT stack is critical for efficient implementation.
Integration with Legacy Systems:
Bridging the gap between traditional IT infrastructures and modern AI-driven tools presents a technical challenge. Seamless integration with legacy systems is vital.
Vendor Lock-in Risks:
Choosing AIOps platforms that limit flexibility is a major concern. Organizations should prioritize solutions with open standards to adapt to evolving needs.
Actionable Insights Generation:
While AIOps can identify root causes and reduce false positives, converting insights into actionable strategies remains difficult for many organizations.
Cultural Resistance:
Internal resistance from IT teams, driven by fears of job displacement or discomfort with technological changes, is common. A strategic change management approach is required to address this.
Scalability and Adaptability:
AIOps platforms must handle growing data volumes and complexities as organizations expand. Scalability and adaptability are critical to long-term success.
AIOps Solutions
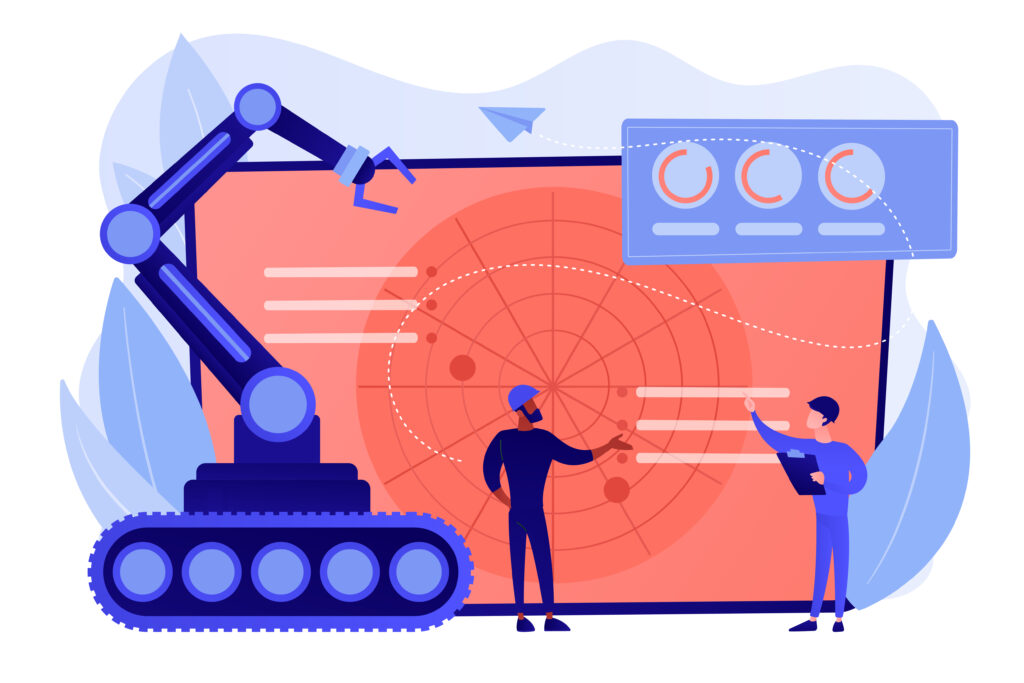
Addressing the Skills and Talent Gap:
Organizations should establish robust training programs tailored to AIOps capabilities, emphasizing continuous learning and skill enhancement. Structured upskilling initiatives can help IT professionals transition from traditional roles to AI-driven management. This can include investments in certification programs, partnerships with external training providers, and fostering internal knowledge-sharing through dedicated platforms.
Improving Data Quality and Integration:
Mitigating Security Risks:
Simplifying Complex IT Infrastructure:
Adopt modular and adaptable architectures to integrate diverse technological environments seamlessly. Microservices, containerization, and API-driven strategies allow platforms to handle complex, multi-layered IT ecosystems effectively.
Managing Data Volumes:
Enhancing Anomaly Detection:
Combine machine learning models with human expertise through hybrid intelligence approaches. This involves designing algorithms that incorporate human insights, minimizing false alerts, and improving contextual understanding of anomalies.
Managing Budgets and Costs:
Adopt a phased approach, starting with pilot implementations that target high-impact areas. Demonstrating measurable ROI through smaller-scale projects can justify broader investments and ensure cost-effectiveness.
Reducing Tool Sprawl:
Streamline IT operations by consolidating multiple functionalities into integrated AIOps platforms. Evaluating existing tools, removing redundancies, and selecting platforms with comprehensive capabilities will simplify the IT toolchain.
Integrating Legacy Systems:
Develop middleware and adaptive layers to bridge the gap between legacy systems and modern AIOps platforms. Flexible integration frameworks are essential for ensuring compatibility and seamless data flow across generations of technology.
Preventing Vendor Lock-in:
Choose AIOps platforms that support open standards, modular architectures, and extensive customization options. Platforms with robust APIs and migration-friendly designs enable adaptability to evolving requirements.
Generating Actionable Insights:
Adopt advanced analytics frameworks to convert machine-generated data into actionable strategies. Visualization tools, contextual interpretation mechanisms, and clear decision-making protocols are crucial for maximizing insights.
Fostering Cultural Transformation:
Ensuring Scalability and Adaptability:
10 Best Practices for Implementing AIOps
Align AIOps with Business Goals
Develop a Robust Data Management Strategy
Customize Machine Learning Algorithms
Implement Phased Roadmap
Adopt an incremental approach to AIOps implementation by starting with targeted pilot programs. Define clear milestones, timelines, and measurable objectives to ensure continuous progress. This phased roadmap allows for gradual scaling, frequent iteration, and refinement of strategies, minimizing disruptions while building confidence in the AIOps solution.
Prioritize Data Security
Make data security a core focus when implementing AIOps. Establish comprehensive security protocols, including strong encryption, advanced access controls, and regular security assessments to mitigate risks. Ensuring compliance with relevant regulations and maintaining the integrity of data within AIOps systems is crucial for protecting sensitive organizational information.
Reduce Alert Noise
Optimize incident management through intelligent alert filtering and management. Key strategies include:
A: Advanced correlation mechanisms to identify critical alerts.
B:Suppressing false alarms to reduce noise.
C:Developing predefined workflows to streamline remediation processes.
D:Implementing intelligent filtering systems that prioritize the most relevant alerts to reduce operational burden.
Foster Cross-Departmental Collaboration
Select Appropriate Tools
Carefully choose AIOps platforms based on critical capabilities such as:
A:Robust data integration features that allow seamless communication between systems.
B:Advanced machine learning support for smarter decision-making.
C:Scalability to accommodate growing operational needs.
Compatibility with existing technological ecosystems to ensure smooth integration with legacy systems and other IT solutions.
Establish Continuous Monitoring
Implement continuous evaluation and monitoring processes to ensure AIOps success. This includes:
A: Regularly measuring performance against defined KPIs.
B: Creating dynamic feedback loops to adapt and improve.
C: Continuously refining AIOps strategies based on real-time performance and operational feedback.
Build Adaptive Learning Capabilities
Develop systems that can learn from historical data, enhance predictive capabilities, and adapt to evolving technological landscapes. Creating adaptive learning frameworks ensures that AIOps becomes increasingly sophisticated over time, providing better insights and more accurate predictions as data accumulates.
By following these best practices, organizations can overcome challenges associated with AIOps adoption and implement a robust, intelligent IT operations framework that supports long-term growth and operational excellence.
[Want to learn more about AIOps challenges and solutions? Click here to reach us.]
Conclusion
In conclusion, while the implementation of AIOps offers immense potential for optimizing IT operations, organizations face several significant challenges along the way. From overcoming the skills and talent gap to managing vast volumes of data, integrating legacy systems, and addressing security concerns, the road to successful AIOps adoption is not without its obstacles.
However, by following best practices such as aligning AIOps initiatives with business goals, developing robust data management strategies, and fostering cross-departmental collaboration, companies can effectively navigate these challenges. With the right tools, a phased approach to implementation, and a strong focus on continuous learning and adaptability, businesses can harness the full power of AIOps to drive smarter, more efficient IT operations.
For organizations looking for expert guidance and robust AI-driven solutions, Bobcares offers comprehensive AI support and development services. With a team of experienced professionals, Bobcares can help navigate the complexities of AIOps, providing tailored solutions that ensure successful implementation and ongoing optimization.
0 Comments