What is Artificial Intelligence for IT Operations And How is It Transforming IT Operations?
AI Ops (Artificial Intelligence for IT Operations) is redefining the way they manage and run their IT business. This cutting-edge integration of artificial intelligence for IT operations allows organizations to navigate the growing complexities of IT infrastructures while ensuring scalability, efficiency, and reliability. Leveraging AI-driven IT management, IT automation, and predictive analytics in IT, AI Ops delivers intelligent solutions for managing incidents, enhancing performance, and reducing downtime.
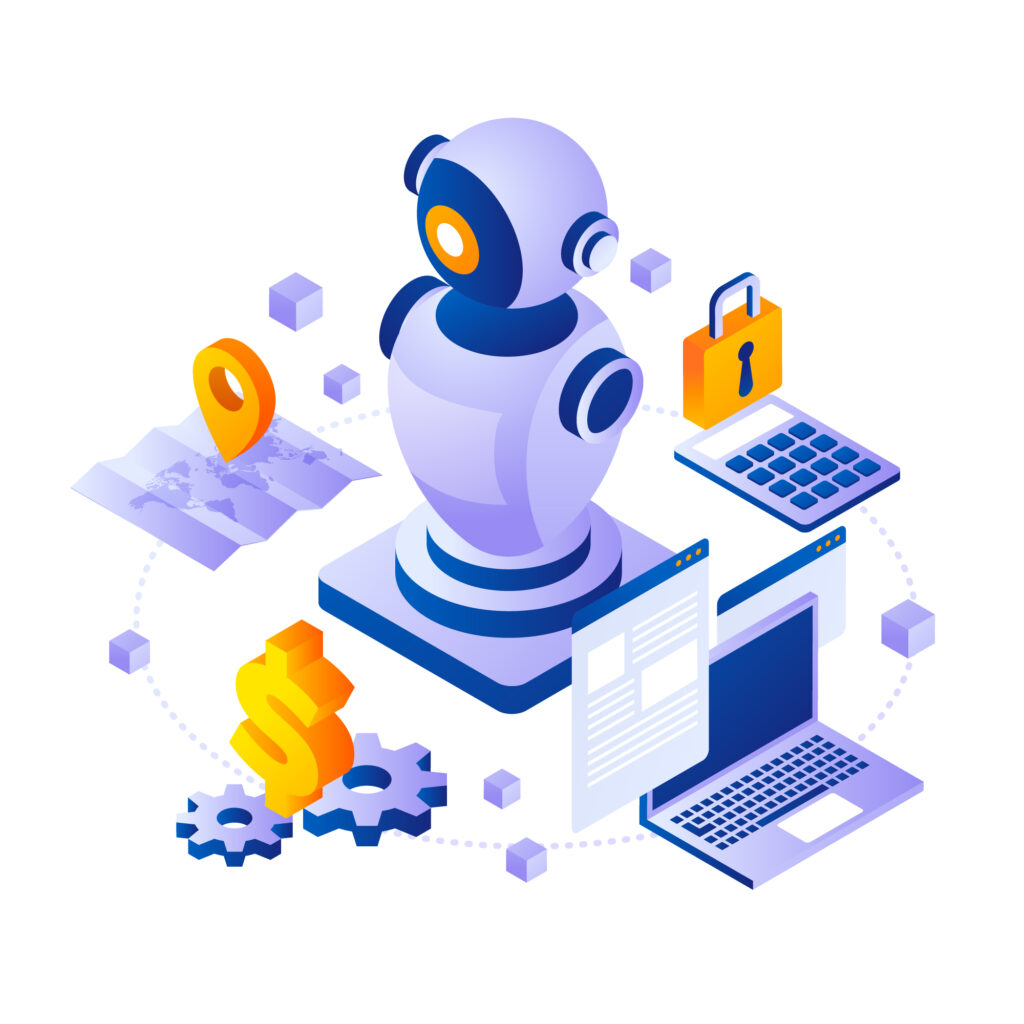
AI Ops emerged as a revolutionary concept in IT management, first introduced by Gartner in 2016. Initially known as “Algorithmic IT Operations,” it evolved into Artificial Intelligence for IT Operations to reflect its broader scope. Its primary aim is to address the increasing intricacies of distributed systems, ensuring intelligent and proactive IT operations. Traditional IT operations relied heavily on manual efforts:
- IT teams manually supervised systems and processes.
- Troubleshooting occurred reactively, only after issues emerged.
- Human intervention was required to resolve problems.
- These processes were slow, error-prone, and resource-intensive.
The evolution of AI-driven IT management was fueled by:
- The explosion of data volumes generated by modern systems.
- The rapid advancements in machine learning technologies.
- The growing complexity of hybrid and multi-cloud infrastructures.
- The need for intelligent and predictive analytics in IT for proactive issue management.
Advantages Over Manual IT Operations
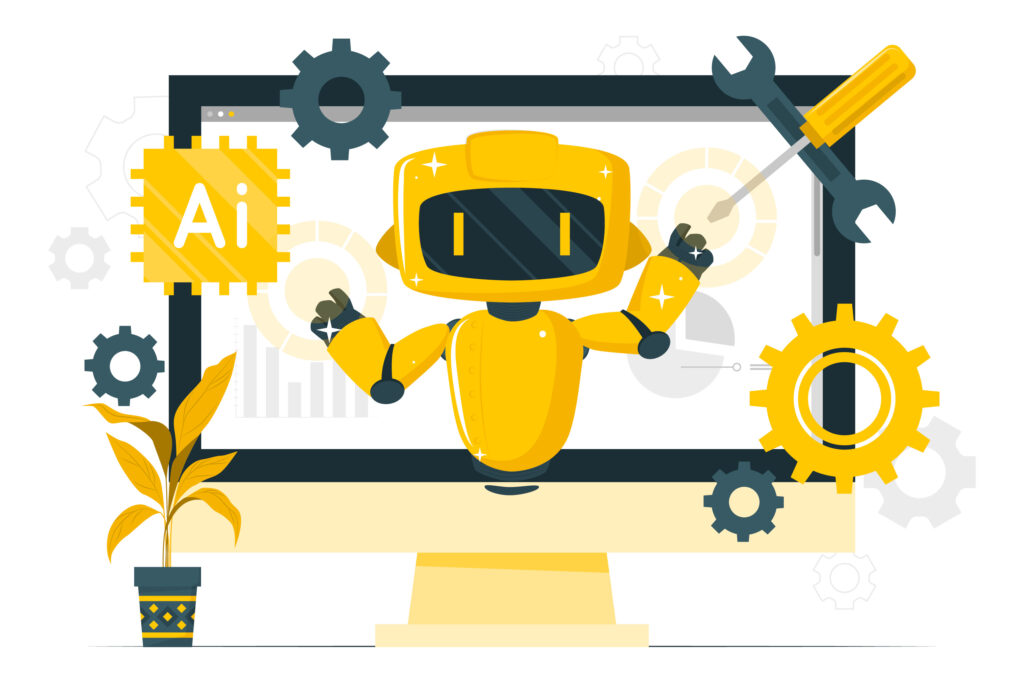
1. Automated Data Processing
AI Ops revolutionizes IT operations by automating and streamlining data management processes. The use of advanced technologies like machine learning algorithms enables organizations to handle data more efficiently and effectively. Here’s how AI Ops enhances automated data processing:
- Real-Time Data Analysis: AI Ops can analyze vast volumes of machine and network data in real-time, allowing IT teams to gain immediate insights into system performance and potential issues. This capability minimizes delays in identifying anomalies and ensures timely responses to emerging challenges.
- Pattern Recognition and Trend Analysis: Leveraging advanced machine learning algorithms, AI Ops identifies patterns and trends within the data. For example, it can detect recurring issues, predict potential failures, and suggest proactive measures to mitigate risks. This insight-driven approach helps organizations stay ahead of potential disruptions.
- Noise Reduction and Event Prioritization: In IT operations, a significant amount of data may not be actionable or relevant. AI Ops filters out irrelevant operational noise, focusing on critical events that require attention. By highlighting what truly matters, it prevents alert fatigue and ensures IT teams can concentrate on impactful tasks.
- Massive Data Processing at Scale: AI Ops processes data at a scale and speed far beyond human capabilities. Whether it’s analyzing logs from thousands of servers, monitoring network traffic across multiple regions, or correlating performance metrics, AI Ops handles these tasks seamlessly, even in the most complex IT environments.
- Focus on High-Value Activities: By automating tedious, manual data handling, AI Ops frees up IT teams to focus on high-value activities such as strategic planning, system optimization, and innovation. This shift not only improves productivity but also enhances the overall efficiency of IT operations.
2. Predictive Capabilities
Artificial Intelligence for IT Operations leverages predictive analytics to transform IT management. This enables teams to anticipate and resolve potential system issues before they escalate. By proactively addressing risks, organizations can achieve greater reliability and performance in their IT operations. Here’s how AI Ops enhances predictive capabilities:
- Anomaly Detection and Event Correlation: AI Ops uses advanced machine learning algorithms to identify anomalies within vast amounts of data. It automatically correlates these anomalies with related events to uncover hidden patterns, revealing early signs of potential system failures. This helps IT teams understand underlying issues and take timely corrective actions.
- Proactive Problem Detection: Through AI-driven IT management, AI Ops goes beyond reactive troubleshooting. It continuously monitors systems, identifying vulnerabilities and predicting issues based on historical and real-time data. This proactive approach reduces downtime and ensures systems operate at optimal performance levels.
Outage Prevention Through Failure Forecasting: Costly outages can significantly impact business operations. AI Ops mitigates this risk by forecasting potential failures. For instance, it can predict when hardware components might degrade or when system loads could exceed capacity, enabling teams to implement preventive measures well in advance.
- Context-Based Insights with Machine Learning: By analyzing data in context, AI Ops provides deeper insights that are actionable and relevant. For example, it can differentiate between critical issues and routine fluctuations, ensuring that IT teams prioritize the right problems and allocate resources efficiently.
- Enhanced System Reliability and Performance: The predictive capabilities of AI Ops significantly enhance the reliability of IT systems. By addressing issues proactively, organizations can maintain seamless operations, improve user experiences, and minimize the risk of disruptions.
3. Enhanced Efficiency
AI Ops revolutionizes IT operations by significantly improving efficiency through automation and intelligent workflows. By handling repetitive and time-consuming tasks, AI Ops empowers IT teams to focus on higher-value, strategic initiatives.
- Automating Network Performance Monitoring and Reporting: AI Ops leverages advanced algorithms to automate the monitoring of network performance in real-time. It collects, analyzes, and reports on key performance metrics without requiring manual intervention. This ensures consistent monitoring and timely identification of performance bottlenecks, allowing IT teams to address issues proactively.
Streamlining Incident Resolution with Intelligent Workflows: When incidents occur, AI Ops employs intelligent workflows to prioritize and resolve them quickly. By automating processes such as root cause analysis and incident categorization, AI Ops reduces the time taken to restore normal operations. For instance, it can escalate critical issues to the right teams and suggest resolutions based on historical data, ensuring faster turnaround times.
- Minimizing Human Intervention: Through task automation, AI Ops reduces the need for repetitive manual tasks, such as updating logs or running diagnostics. By offloading these duties to AI-driven systems, IT teams can concentrate on strategic initiatives like system upgrades, innovation, and long-term planning.
- Optimized Resource Allocation: AI Ops intelligently allocates IT resources based on data-driven insights. For example, it can predict workload spikes and adjust server capacity accordingly, ensuring efficient use of infrastructure. This reduces resource wastage and enhances cost efficiency.
- Faster Response Times: Moreover, the automation capabilities of AI Ops ensure quicker responses to potential issues, effectively preventing minor problems from escalating into major disruptions. This agility, in turn, is critical for maintaining business continuity and reducing downtime.
- Improved Operational Reliability: By handling tasks with precision and consistency, AI Ops minimizes errors caused by manual operations. This leads to greater reliability in IT processes and builds trust in system performance.
4. Comprehensive Monitoring
Artificial Intelligence for IT Operations redefines IT monitoring by offering a unified, real-time view of complex environments, ensuring seamless oversight and proactive management. Its comprehensive approach addresses the challenges of modern hybrid and multi-cloud infrastructures. Here’s how AI Ops achieves this:
- Unified Monitoring Across Hybrid and Multi-Cloud Infrastructures: AI Ops excels at monitoring diverse IT environments, including on-premise systems, hybrid setups, and multi-cloud architectures. It integrates data from various sources to provide a single, cohesive view of the entire infrastructure.
- Automatic Visibility into Asset Dependencies: Modern IT systems often involve interconnected assets and applications, making manual dependency mapping complex and error-prone. AI Ops eliminates the need for human oversight by automatically detecting and mapping these dependencies. This enables IT teams to understand how issues in one area might impact other parts of the system.
- Centralized Dashboard for Actionable Insights: AI Ops consolidates data from various monitoring tools and sources into a centralized dashboard. This dashboard not only displays real-time performance metrics but also highlights anomalies, trends, and actionable insights.
- End-to-End Observability: AI Ops ensures observability across the entire IT stack, from infrastructure and applications to network performance. This end-to-end visibility helps detect and resolve issues before they impact users, enhancing system reliability and customer satisfaction.
- Real-Time Alerts and Notifications: AI Ops monitors systems continuously, sending real-time alerts when anomalies or potential issues are detected. These alerts are often enriched with context, such as the probable cause and suggested resolutions, enabling IT teams to respond promptly and effectively.
- Scalability and Adaptability: Furthermore, as IT environments grow in complexity, AI Ops scales effortlessly to accommodate additional systems and infrastructure. It also adapts to evolving business needs, ensuring that monitoring capabilities remain both robust and effective.
5. Continuous Learning
AI Ops platforms harness the power of continuous learning to stay adaptive and effective in dynamic IT environments. By integrating advanced adaptive learning mechanisms, these platforms evolve to meet the growing complexity and demands of IT operations. Here’s how they achieve this:
- Real-Time Data Collection for Model Refinement: AI Ops platforms continuously collect operational data in real-time from diverse IT systems, including servers, networks, and applications. This constant influx of fresh data enables the refinement of predictive models, ensuring they stay relevant and accurate. The more data the system processes, the better it becomes at identifying patterns and predicting outcomes.
- Advanced Machine Learning for Evolving Analytics: At the core of continuous learning is the use of sophisticated machine learning algorithms. These algorithms adapt to changing conditions and new data, allowing AI Ops platforms to detect previously unseen patterns or anomalies.
- Enhanced Predictive Accuracy: Moreover, through iterative learning and processing, AI Ops platforms significantly enhance the accuracy of their predictions. In addition, continuous data analysis helps fine-tune algorithms, thereby reducing false positives and negatives in anomaly detection.
- Self-Optimizing Systems: Furthermore, AI Ops platforms use continuous learning to become self-optimizing. They automatically adjust their thresholds, enhance alert mechanisms, and refine correlation techniques, thereby minimizing the need for manual intervention.
- Building Institutional Knowledge: Moreover, by processing and learning from historical and real-time data, AI Ops builds a robust repository of institutional knowledge. This repository helps the platform anticipate recurring issues, recommend optimal configurations, and provide valuable insights based on past experiences.
- Proactive Insights and Recommendations: Furthermore, the continuous learning capabilities of AI Ops enable it to provide proactive insights and actionable recommendations. As a result, IT teams receive suggestions on optimizing system performance, addressing potential vulnerabilities, and implementing best practices for infrastructure management.
Core Technological Components of AI Ops
Artificial Intelligence for IT Operations relies on a robust technological foundation to deliver AI-driven IT management and IT automation effectively.
1. Data Collection
AI Ops aggregates diverse data sources, such as:
- Historical logs and real-time metrics.
- Performance traces and application data.
- Incident tickets and network traffic.
This ensures a comprehensive data pool for intelligent analysis.
2. Machine Learning Algorithms
Machine learning forms the backbone of AI Ops, employing techniques such as:
- Supervised Learning: Identifying predefined patterns.
- Unsupervised Learning: Detecting anomalies without predefined labels.
- Reinforcement Learning: Continuously improving through adaptive feedback.
- Deep Learning: Recognizing complex patterns within extensive datasets.
3. Advanced Analytics
AI Ops provides cutting-edge analytics capabilities:
- Correlating events in real-time for effective incident management.
- Detecting performance degradation and conducting root cause analysis.
- Offering actionable insights for proactive decision-making.
4. Automation and Orchestration
IT automation in Artificial Intelligence for IT Operations enables:
- Automatic resource scaling based on demand forecasts.
- Intelligent incident routing for quicker resolutions.
- Executing predefined remediation scripts to resolve known issues autonomously.
5. Continuous Learning and Adaptation
AI Ops systems enhance operational effectiveness by:
- Adapting to changing IT environments.
- Refining responses based on historical data.
- Incorporating predictive analytics in IT to improve future outcomes.
Key Features of AI Ops
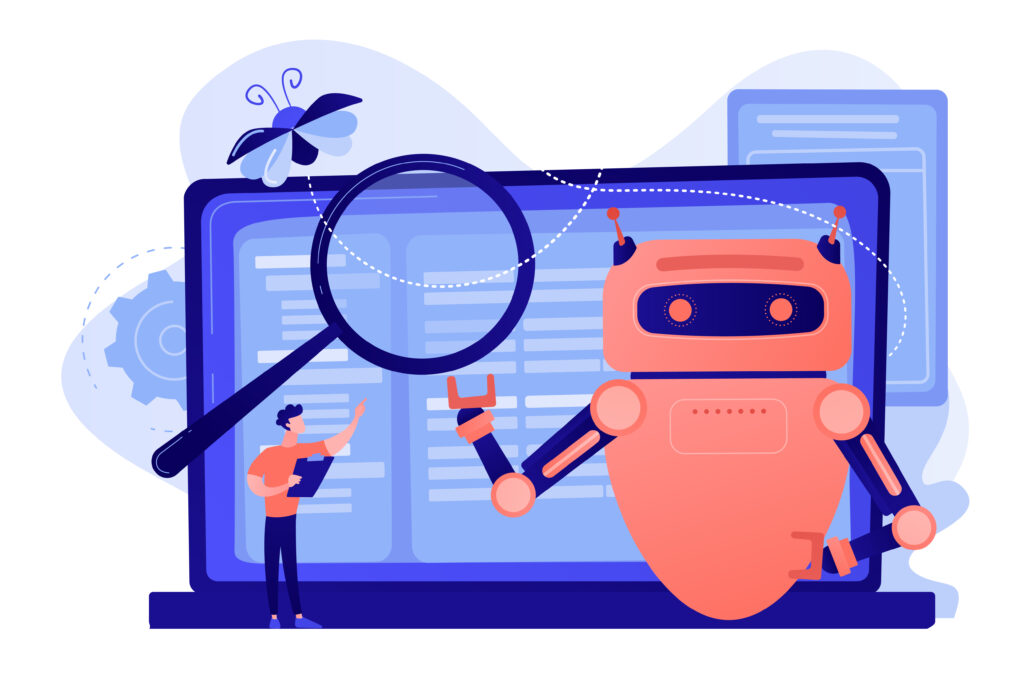
Anomaly Detection
Artificial Intelligence for IT Operations employs AI-driven IT management techniques to detect anomalies:
- Analyzing data from logs, metrics, and network traffic for unusual patterns.
- Prioritizing events based on potential business impacts.
- Leveraging machine learning algorithms to minimize false positives.
Techniques such as Isolation Forest and Local Outlier Factor (LOF) enable efficient identification of irregularities in high-dimensional data.
Predictive Analytics
Predictive analytics in IT enables proactive problem-solving by:
- Analyzing historical and real-time data trends.
- Anticipating system bottlenecks and vulnerabilities.
- Ensuring dynamic resource allocation based on future requirements.
This allows IT teams to prevent disruptions before they occur.
Applications of AI Ops: Transforming IT Operations
Incident Management and Resolution
AI Ops revolutionizes incident management by:
- Detecting incidents through comprehensive data analysis.
- Utilizing AI automation for routine issue resolution.
- Prioritizing incidents based on severity for faster response times.
Optimizing IT Infrastructure Performance
Through continuous monitoring, AI Ops:
- Tracks CPU usage, memory, and network bandwidth in real-time.
- Predicts future resource needs, ensuring proactive capacity planning.
- Distributes workloads dynamically to prevent performance issues.
Challenges in AI Ops Implementation
Data Integration Challenges
- Fragmented data silos limit cross-functional collaboration.
- Inconsistent data formats create obstacles for AI systems.
False Positives and Negatives
- Establishing precise thresholds for anomaly detection remains a challenge.
- Excessive alerts can hinder the efficiency of IT teams.
Technical Complexity
- Sophisticated AI integrations require advanced infrastructures and expertise.
- Many organizations face resource constraints during initial implementations.
Future of AI Ops: The Road Ahead
The future of AI-driven IT management lies in:
- Enhanced integration of edge computing and 5G networks for real-time processing.
- Proactive infrastructure management through predictive analytics in IT.
- Intelligent, self-healing systems that minimize operational disruptions.
By incorporating AI Ops, businesses can transform their IT operations into intelligent.
[Want to learn more about Artificial Intelligence for IT Operations? Click here to reach us.]
Conclusion
AI Ops is transforming IT operations in 2024 by leveraging AI, machine learning, and automation to streamline tasks, enhance system reliability, and improve efficiency. From automated data processing and predictive capabilities to comprehensive monitoring and continuous learning, AI Ops helps IT teams manage complex environments more effectively. By proactively addressing issues before they arise, AI Ops reduces downtime and optimizes resource allocation.
As organizations adopt hybrid and multi-cloud infrastructures, AI Ops becomes essential for maintaining performance and minimizing operational costs. Partnering with experts like Bobcares, who specialize in AI development services, helps businesses integrate AI-driven solutions seamlessly, ensuring optimal results.
Embracing AI Ops enables businesses to move from reactive to proactive IT management, allowing IT teams to focus on strategic growth and innovation in a rapidly evolving digital world.
0 Comments